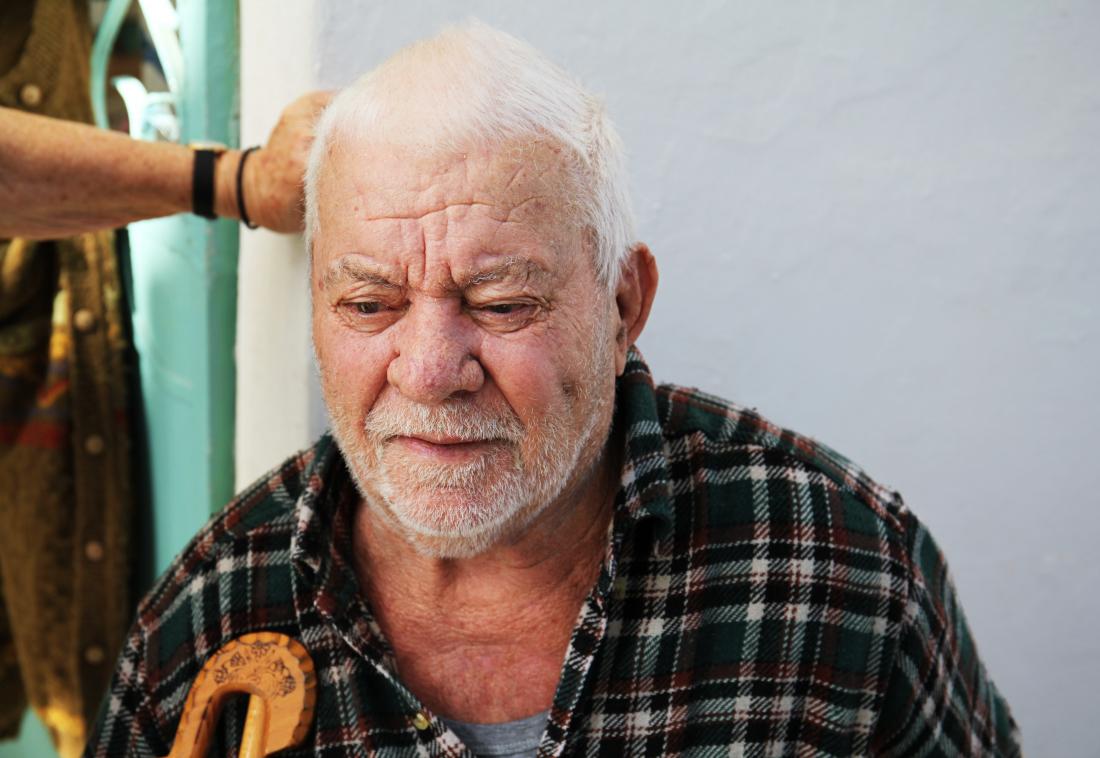
MIT researchers have developed a machine-learning model that they say could accurately predict cognitive decline.
Alzheimer’s disease affects millions of people worldwide, yet scientists still do not know what causes it.
For this reason, prevention strategies can be hit and miss. Moreover, healthcare professionals have no clear way of determining a person’s rate of cognitive decline once a doctor has diagnosed them with Alzheimer’s.
Now, researchers from the Massachusetts Institute of Technology (MIT) in Cambridge — in collaboration with specialists from other institutions — have developed a machine-learning model that could allow specialists to predict how much a person’s cognitive functioning will change up to 2 years in advance of this decline becoming established.
The team — made of Ognjen Rudovic, Yuria Utsumi, Kelly Peterson, Ricardo Guerrero, Daniel Rueckert, and Prof. Rosalind Picard — will present their project later this week at the Machine Learning for Healthcare conference. This year’s conference will take place in Ann Arbor, MI.
“Accurate prediction of cognitive decline from 6 to 24 months is critical to designing clinical trials,” Rudovic explains. This, he adds, is because “[b]eing able to accurately predict future cognitive changes can reduce the number of visits the participant has to make, which can be expensive and time consuming.”
“Apart from helping develop a useful drug,” the researcher continues, “the goal is to help reduce the costs of clinical trials to make them more affordable and done on larger scales.”
Using meta learning to predict decline
In order to develop their new model, the team used data from the Alzheimer’s Disease Neuroimaging Initiative (ADNI), which is the largest Alzheimer’s disease clinical trial dataset in the world.
Through ADNI, the researchers were able to access the data of approximately 1,700 people — some with and some without Alzheimer’s disease — collected over 10 years.
The team had access to clinical information, including participants’ cognitive functioning assessments, brain scans, data regarding individuals’ DNA makeup, and cerebrospinal fluid measurements, which reveal Alzheimer’s disease biomarkers.
As a first step, the researchers developed and tested their machine-learning model using data from a subgroup of 100 participants. However, there were a lot of missing data about this cohort. So, the investigators decided to use a different statistic approach to analyze the cohort’s available data in a way that would make the analysis more accurate.
Still, the new model did not reach the level of accuracy that its developers had expected. To render it even more accurate, the researchers used data from another subcohort of ADNI participants.
This time, however, the team decided against applying the same model to everyone. Instead, they personalized the model to fit each participant, taking in new data as they became available after each new clinical assessment.
With this approach, the researchers found that the model led to a significantly lower error rate in its predictions. Moreover, it performed better than existing machine-learning models applied to clinical data.
Still, the researchers went a step further to make sure that their approach left room for as little error as possible. They went on do devise a “meta learning” model that can pick the best approach to predict cognitive outcomes in each participant.
This model automatically chooses between the overall population and the personalized approach, calculating which one will most likely offer the best prediction for any given individual at a particular point in time.
The researchers found that this approach reduced the error rate for predictions by as much as an additional 50%.
“We couldn’t find a single model or fixed combination of models that could give us the best prediction,” explains Rudovic.
“So we wanted to learn how to learn with this meta learning scheme. It’s like a model on top of a model that acts as a selector, trained using meta knowledge to decide which model is better to deploy.”
Ognjen Rudovic
Going forward, the team aims to form a partnership with a pharmaceutical company to test this model in an ongoing Alzheimer’s disease trial.